FaceEngage
The Problem:
Measuring user engagement in interactive tasks can facilitate numerous applications toward optimizing user experience, ranging from eLearning to gaming. However, a significant challenge is the lack of non-contact engagement estimation methods that are robust in unconstrained environments.
FaceEngage: Robust Estimation of Gameplay Engagement from User-contributed (YouTube) Videos
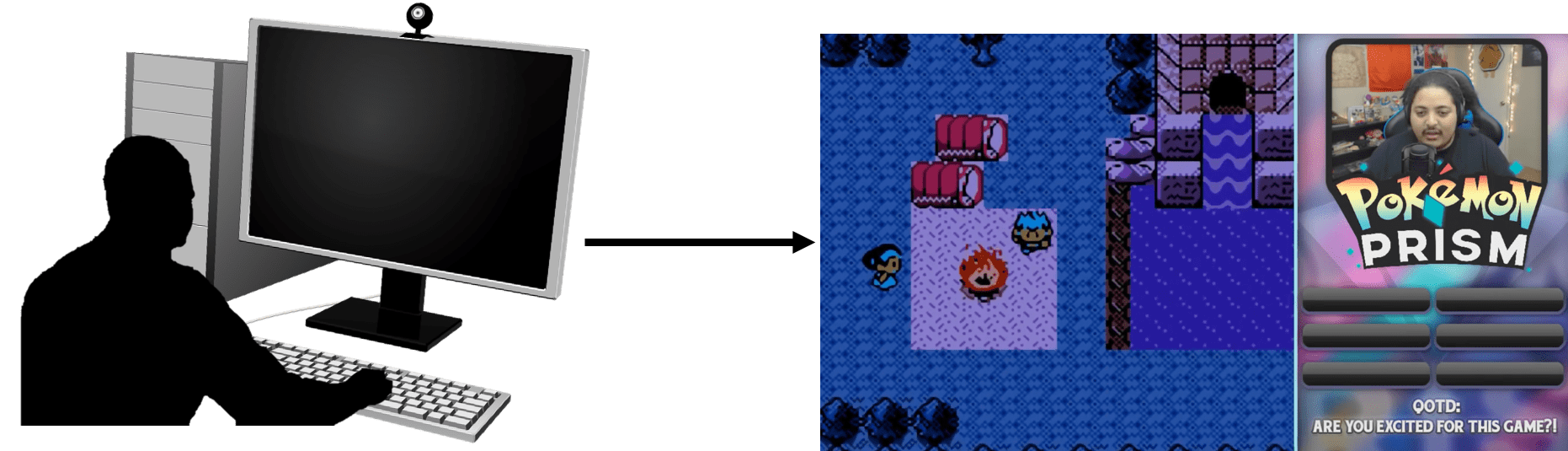
Our Solution:
We present FaceEngage, a non-intrusive engagement estimator leveraging user facial recordings during actual gameplay in naturalistic conditions. Our contributions are three-fold. First, we show the potential of using front-facing videos as training data to build the engagement estimator. We compile FaceEngage Dataset with over 700 picture-in-picture, realistic, and user-contributed YouTube gaming videos (i.e., with both full-screen game scenes and time-synchronized user facial recordings in subwindows). Second, we develop FaceEngage system, that captures relevant gamer facial features from front-facing recordings to infer task engagement. We implement two FaceEngage pipelines: an estimator trained on user facial motion features inspired by prior psychological works, and a deep learning-enabled estimator. Lastly, we conduct extensive experiments and conclude: (i) certain user facial motion cues (e.g., blink rates, head movements) are engagement-indicative; (ii) our deep learning-enabled FaceEngage pipeline can automatically extract more informative features, outperforming the facial motion feature-based pipeline; (iii) FaceEngage is robust to various video lengths, users/game genres and interpretable. Despite the challenging nature of realistic videos, FaceEngage attains the accuracy of 83.8% and leave-one-user-out precision of 79.9%, both of which are superior to our face motion-based model.
Team
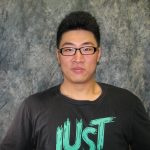
Xu Chen
Graduate Student
ECE, Rice University
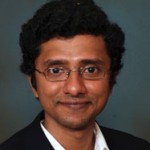
Dr. Ashok Veeraraghavan
Associate Professor
ECE, Rice University
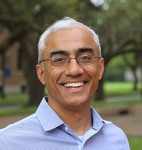
Dr. Ashutosh Sabharwal,
Professor
ECE, Rice University
Paper
Xu Chen, Li Niu, Ashok Veeraraghavan, and Ashutosh Sabharwal, FaceEngage: Robust Estimation of Gameplay Engagement from User-contributed (YouTube) Videos. IEEE Transactions on Affective Computing (2019)